Options
An Exploratory Comparison of Stock Price Prediction: Using Multiple Machine Learning Approaches based on Global Stock Indices
Journal
Palgrave Studies of Entrepreneurship and Social Challenges in Developing Economies
Entrepreneurship, Innovation, and Technology
Date Issued
2024-11-29
Author(s)
Chin Yang Lin
DOI
doi.org/10.1007/978-3-031-65314-8_14
Abstract
Predicting stock prices is difficult because of their multiple input variables, volatility, and unpredictable nature. To provide a suitable model for forecasting the global stock market, this study conducts an exploratory analysis comparing two models based on Artificial Intelligence: Support Vector Machine (SVM) and Long Short-Term Memory (LSTM) Neural Networks. The work considers a publicly accessible dataset and uses feature engineering to extract time-series features. Stock price predictions are made using the SVM and LSTM algorithms. For this purpose, Accuracy (ACC) and Root Mean Squared Error (RMSE) are considered accuracy and performance measures. According to the results, LSTM with mean accuracy (ACC) = 0.9061 achieved better accuracy than SVM with mean accuracy (ACC) = 0.881. SVM with mean RMSE = 0.729 achieved better performance and the degree of fit to the data than LSTM with mean RMSE = 427.1. According to the results, the study demonstrates the effectiveness and applicability of machine learning methods for estimating the values of the global stock market and providing valuable models for researchers, analysts, and investors.
File(s)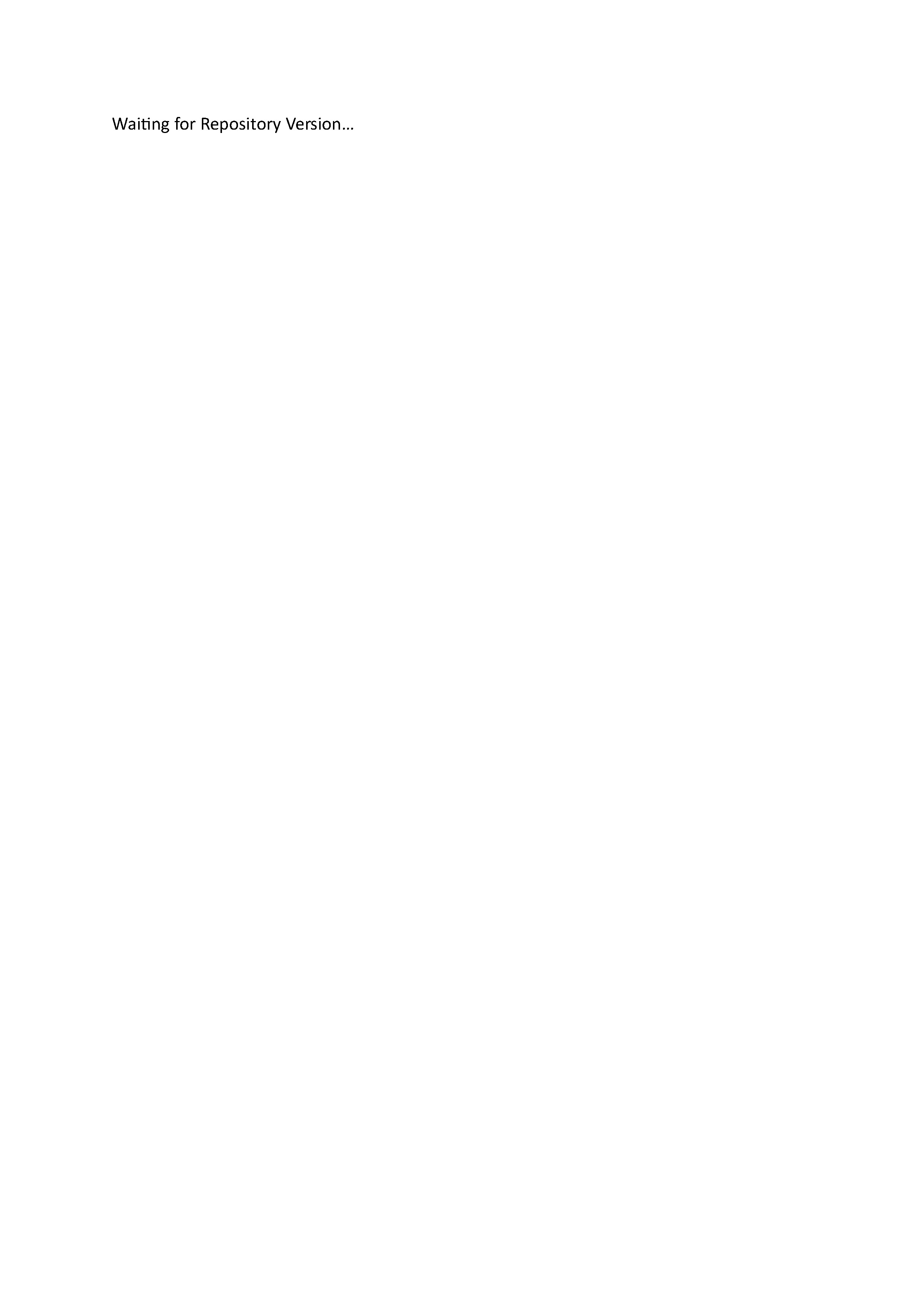
Loading...
Name
Waiting for Repository Version.jpg
Size
113.46 KB
Format
JPEG
Checksum
(MD5):45228bea27122db72e82ca06d42d0a3e